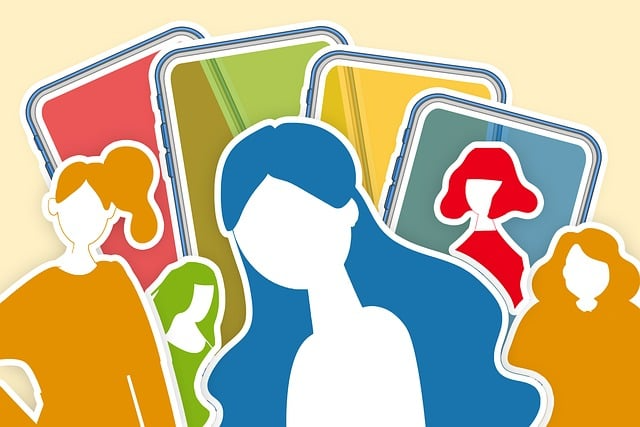
A/B Testing with AI: Optimizing Social Media Ad Campaigns
In the ever-evolving world of social media advertising, A/B testing has been a cornerstone in understanding consumer preferences and optimizing ad performance. This method compares two versions of an ad to see which performs better. With the advent of Artificial Intelligence (AI), A/B testing is undergoing a transformative shift. AI brings the power to analyze vast datasets rapidly, turning traditional A/B testing into a sophisticated, predictive tool.
This integration is revolutionizing how social media ad campaigns are optimized, making them smarter and more effective. Our exploration focuses on how AI-driven A/B testing can outperform traditional methods, marking a new era in digital marketing strategy.
Discover the power of AI-driven A/B testing in social media advertising with Crescitaly, the best SMM Panel since 2012. Elevate your Facebook, Instagram, Youtube, Telegram, and Twitter services. Explore more on Crescitaly.com for cutting-edge marketing solutions.
Basics of A/B Testing
A/B testing, at its core, is a methodological approach used to compare two versions of a web page or ad to determine which one performs better. In the context of social media advertising, this typically involves presenting two variants of an ad (labelled as A and B) to a similar audience and analyzing which version drives more engagement or conversions. This method has been instrumental in guiding advertisers towards decisions that resonate more effectively with their target audience.
Traditionally, A/B testing in social media advertising has been a largely manual process. It involves creating two distinct versions of an ad—each differing in specific elements like headlines, images, or call-to-action phrases—and then running these ads simultaneously to measure their performance. The primary limitation of this traditional approach is its reliance on linear and often time-consuming processes. Marketers have to wait for a significant amount of data to accumulate before they can make a confident decision, which might delay the optimization of ad campaigns.
The key metrics used in A/B testing for social media ads are vital in determining the success of each version. These include:
- Click-Through Rate (CTR): This measures the percentage of clicks an ad receives relative to its total impressions, indicating how compelling the ad is in driving user interaction.
- Conversion Rate: This metric assesses the percentage of users who take a desired action after clicking on the ad, like making a purchase or signing up for a newsletter, reflecting the ad's effectiveness in converting interest into action.
- Engagement Rate: Often specific to social media, this rate evaluates how users interact with the ad through likes, shares, comments, etc., providing insights into how engaging and relevant the ad content is to the audience.
The Advent of AI in A/B Testing
The integration of Artificial Intelligence (AI) in A/B testing represents a significant paradigm shift, offering a more advanced, nuanced approach to optimizing social media ad campaigns. At the heart of this integration are technologies like machine learning and predictive analytics, which are changing the landscape of digital marketing.
Machine Learning in A/B Testing:
Machine learning, a subset of AI, involves algorithms that learn from and make predictions based on data. In A/B testing, machine learning algorithms can analyze historical and real-time data to identify patterns and trends that human analysts might miss. This capability enables a more nuanced understanding of consumer behavior and preferences.
Predictive Analytics:
This aspect of AI involves using data, statistical algorithms, and machine learning techniques to identify the likelihood of future outcomes based on historical data. In the context of A/B testing, predictive analytics can forecast which ad variant is likely to perform better, even before the test is fully completed. This leads to quicker, more informed decision-making.
Efficiency in Analyzing Complex Data Sets:
One of the most significant advantages of AI in A/B testing is its ability to efficiently process and analyze large, complex data sets. AI algorithms can quickly sift through layers of data - including demographics, user behavior, and engagement metrics - to derive actionable insights. This efficiency not only speeds up the A/B testing process but also enhances the accuracy of the insights gained.
Examples of AI in Action:
There have been several instances where AI-driven A/B tests have yielded remarkable results. For example, an online retailer might use AI to analyze customer data and predict which type of product images (lifestyle vs. plain background) would lead to higher engagement and sales. Similarly, a streaming service could employ AI algorithms to determine which thumbnail variations for a show would increase play rates.
Optimizing Ad Campaigns with AI-Driven A/B Testing
The integration of Artificial Intelligence (AI) into A/B testing has redefined the process of optimizing social media ad campaigns. AI enhances each stage of the A/B testing cycle, from hypothesis generation to data analysis, offering numerous benefits while also presenting certain challenges.
Enhancing the A/B Testing Process:
- Hypothesis Generation: AI can analyze past campaign data to generate informed hypotheses. For example, machine learning models can identify which ad elements (colors, messaging, layout) have historically performed well with certain audience segments, thereby guiding the creation of more targeted A/B test variants.
- Variable Selection: AI algorithms can assist in selecting the most impactful variables for testing. By analyzing large datasets, AI can identify subtle but significant variables that might be overlooked in manual processes, such as specific time slots, user demographics, or even weather conditions.
- Data Analysis: AI excels in swiftly analyzing the results of A/B tests. It can process complex datasets to identify not just which variant performed better, but also why it performed better. This deep analysis can provide insights that go beyond simple performance metrics, offering a more nuanced understanding of consumer behavior.
Benefits of Using AI in A/B Testing:
- Increased Accuracy: AI's ability to process vast amounts of data with sophisticated algorithms leads to more accurate predictions and insights, reducing the likelihood of human error.
- Faster Results: AI-driven analysis can rapidly process data, providing real-time insights that enable quicker decision-making. This speed is crucial in the fast-paced world of social media marketing.
- Scalability: AI systems can easily handle large-scale A/B tests across multiple campaigns and platforms, something that would be resource-intensive and time-consuming if done manually.
Challenges and Considerations:
- Data Privacy Concerns: As AI systems require access to large amounts of data, there are concerns regarding user privacy and data protection. Ensuring compliance with data privacy regulations like GDPR is crucial.
- AI Bias: If the training data for AI models is biased, the AI's decisions and predictions will be biased too. This necessitates careful consideration and continuous monitoring to ensure that the AI's learning process is as unbiased as possible.
Future Trends in AI and A/B Testing
As Artificial Intelligence (AI) continues to evolve, its impact on A/B testing and, by extension, social media advertising is expected to deepen and diversify. This evolution is not only shaping current practices but also paving the way for future trends and developments that could further revolutionize the field.
Emerging Trends and Developments:
- Advanced Predictive Analytics: Future AI systems are likely to offer even more sophisticated predictive analytics capabilities. This might involve predicting consumer behavior changes in response to external factors like market trends or global events, allowing for more proactive and preemptive campaign adjustments.
- Increased Personalization: AI's capacity for handling big data will enable hyper-personalized ad experiences. Machine learning algorithms could tailor content in real-time based on individual user interactions, leading to highly personalized ad campaigns at scale.
- Integration with Other Technologies: The convergence of AI with other emerging technologies like Augmented Reality (AR) and the Internet of Things (IoT) could open up new avenues for interactive and immersive ad experiences, providing richer data for A/B testing.
Predictions for Social Media Advertising:
- Automated Optimization: AI might advance to the point where it can autonomously run and optimize A/B tests, continuously learning and adapting strategies in real-time without much human intervention.
- Behavioral Prediction Models: Future AI could develop more nuanced models that predict consumer behavior with greater accuracy, taking into account a wider range of variables, including emotional responses and psychological patterns.
- Ethical Advertising: With the growing concern about manipulative advertising, AI might be employed to ensure ethical advertising practices, balancing business goals with consumer well-being.
Potential Challenges and Ethical Considerations:
- Data Privacy and Security: As AI technologies become more ingrained in social media advertising, concerns about data privacy and security will intensify. Ensuring ethical use of consumer data and protecting against breaches will be paramount.
- Algorithm Transparency: There will be a growing need for transparency in AI algorithms to prevent biases and ensure fair and ethical use of AI in advertising.
- Regulatory Compliance: As AI in advertising evolves, so will the regulatory landscape. Staying compliant with emerging laws and guidelines will be a continuous challenge for marketers.
Conclusion
The integration of Artificial Intelligence (AI) in A/B testing marks a transformative era in social media advertising. By enhancing decision-making with data-driven insights, AI-driven A/B testing offers a path to more effective and personalized ad campaigns. While it brings numerous benefits, it also introduces challenges like data privacy and AI bias.
Embracing this technology responsibly and ethically is crucial for the future of digital marketing. As AI continues to evolve, it holds the promise of revolutionizing social media advertising strategies, making adaptability and awareness key for marketers in this rapidly changing landscape.